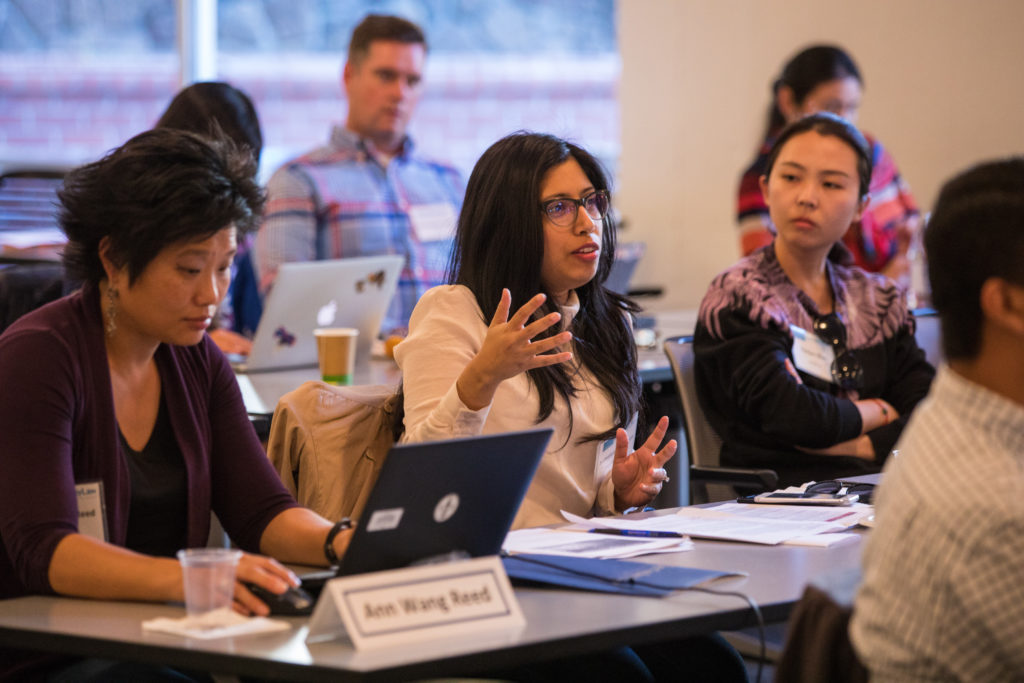
At Artificial Intelligence Unlocked, Berkeley Law Executive Education hosted practitioners from across industries to explore AI and machine learning technologies, industry applications, and legal and policy considerations.
By Rose Ors
Berkeley Law Executive Education Fellow
December 2018
Join us for the next AI Unlocked Academy and earn a certificate in Artificial Intelligence from UC Berkeley!
[button url=”https://executive.law.berkeley.edu/in-person-programs/ai-unlocked/” color=”medalist” target=”_blank”]LEARN MORE[/button]
The artificial intelligence (AI) explosion is a game changer. A recent PwC report estimates that AI could contribute up to $15.7 trillion to the global economy in 2030. The rush by nations to lead the “Fourth Industrial Revolution” has been likened to the space race of the 20th century. So, true to its promise to provide innovative and interactive programs for organizational leaders and legal practitioners, Berkeley Law Executive Education teamed up with the Berkeley Center for Law and Technology (BCLT) last month to offer their first Academy on AI called Artificial Intelligence Unlocked.
The three-day program covered how AI works, its current and future applications, and the legal, social and policy implications of the technology. AI experts in business, academia, and law led the lectures and discussions and guided the attendees on an AI journey filled with a mix of “wow” and “oh no” moments. We were “wowed” by the potential transformative impacts of AI. We said “oh no” to AI’s flaws; flaws that could undermine the trust not only in the technology but the institutions that use it.
AI—Hype & Reality
A highlight of the Academy was a TED-worthy lecture by Ken Goldberg, roboticist and William S. Floyd Distinguished Chair of Engineering at UC Berkeley. Goldberg dismissed the notion that AI will evolve into a super-human intelligence that poses an existential threat to the human race (Singularity), noting that “the utopian and dystopian views of AI are highly distorted.” In his view, robots will not take over the world, nor take vast swaths of jobs from humans. What he does foresee is a future where the diverse skills of humans and machines work together (Multiplicity). He gave a number of examples to elucidate the point, one being the highly publicized 2017 win of Google’s AlphaGo computer program over the world’s top-ranked Go player. The computer’s victory was a significant achievement for machine learning. However, Goldberg explained, what received less notice was far more interesting—the world’s top Go players beat the AlphaGo program when they played the game alongside machines in “human-computer” teams. Harnessing the power of human-machine collaboration, Goldberg argues, is the winning combination in this era of artificial intelligence.
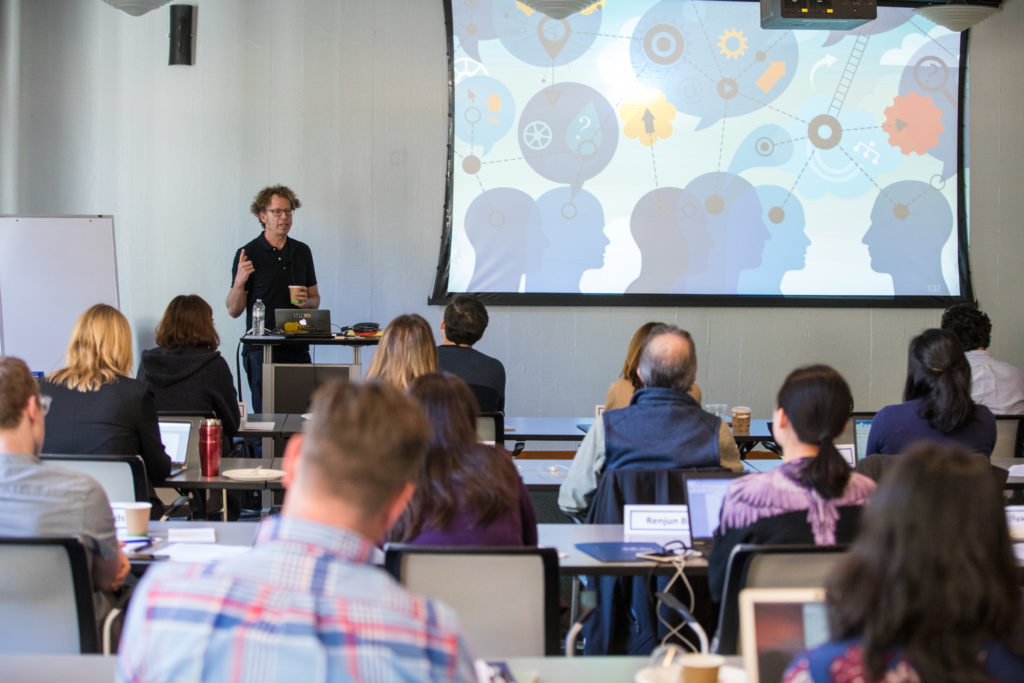
Professor Ken Goldberg explores the future of AI, robotics, and machine learning.
AI—The “Wows” and “Oh, No“ Factors
There were a number of presentations that focused on AI’s transformative impact on industry, governments, and the world. Indeed, it was the global transformative role of AI that was the clarion call of AI academics and industry veterans—including Peter Norvig, Director of Research at Google and author of the leading AI textbook, “Artificial Intelligence: A Modern Approach.” The examples filled the audience with wonder at the many current and in-development AI fueled inventions. The “wow” applications included many that would change how work is done by workers ranging from truck drivers to surgeons. Drawing particular awe were AI projects in medicine that hold out the promise of saving, extending and enhancing human life.
The Role of Data
A stellar lecture by Gregory LaBlanc, Faculty Director at Berkeley Fintech Institute, focused on the essential role data plays in the evolution of AI; most critically in the subset of AI known as machine learning. In LaBlanc’s view, those who hold the most data will win the race in developing machine learning solutions. For example, LaBlanc argued that Tesla has a competitive advantage in developing driverless vehicles because it has been collecting data on driving behavior far longer than its competitors. In the case of China, notes LaBlanc, it can leapfrog other nations in AI development because of its immense population and ability to collect its citizens’ data as it sees fit.
Having explained the power of data, LaBlanc next turned to one of the “oh no’s” of data—bias. To illustrate the point, he drew on examples of data bias that occurs in a subset of machine learning called deep learning. In deep learning, explained LaBlanc, the learning process is highly iterative (as in humans) and requires that an immense amount of data (training data) be fed to the machine so it can detect patterns and from those patterns, extrapolate insights. The examples of biased data underscored the adage of “garbage-in-garbage-out.”
To frame the problem of using biased data in machine decision-making, LaBlanc used a hypothetical HR case: A company’s HR department wants to hire more high performing employees. It feeds its AI applicant screening tool historical and current data on the characteristics of the company’s top performers. The data trains the tool’s screening algorithm to pick candidates who have the same or similar characteristics. If the company’s hiring practices have been to hire and promote mostly white males—let’s say it is a tech company and its hiring more engineers—the algorithm will more than likely “learn” that the best performing engineers are white males and recommend white male candidates. In similar scenarios where gender and/or racial bias is embedded in the data (input), the recommendations (output) are likely to be biased.
So, how can the user of an AI decision tool discover how the technology came up with a biased or otherwise erroneous recommendation? The question is at the center of the concern about AI-based recommendations used to make life-altering decisions in areas such as financial and healthcare services, employment, social services, and the criminal justice system.
The Black Box Problem
The question of “explainability” of AI systems is another “oh no” issue with AI. Today, AI systems with deep-learning neural networks behave like black boxes—what they generate cannot be explained, even by the engineers who created the system. Why? A math-free, shorthand explanation is that the neural networks—the software structure that underlies deep learning—are designed to let the machine learn on its own. It is the machine itself that discovers the most useful patterns to use for a set objective. The problem is so endemic that a whole field of research in AI called “explainability” is trying to tackle the problem.
The joint problem of bias and accountability is one of pressing concern where issues of due process and civil liberties are at stake. Making a case for the eminent nature of the problem was Cindy Cohn, Executive Director of the Electronic Frontier Foundation. Cohn argued that the use of AI by government agencies to help make critical decisions about people’s lives leaves no room for algorithmic mystery. She shared a number of examples where AI is being used to help make decisions in critical stages of the criminal justice system (e.g., policing, bail, sentencing). The examples show how these AI driven decisions have produced biased outcomes. Why? As in LaBlanc’s HR scenario, the crime data fed to the AI tool are embedded in the historical bias of the criminal justice system. So, biased garbage-in, biased garbage out. It is a catch-22 problem.
Legal and Ethical Framework
So, how do we solve the catch-22 problem? The final day of the Academy established that the question is in search of an answer. That said, efforts to solve the problem of bias and explainability are underway. A number of task forces have been launched by advocacy groups, private companies, and governments. However, what can a company that is developing a new AI solution do to prepare itself for charges of bias and lack of explanability?
Lindsey Tonsager, a partner at Covington & Burling, and co-chair of the firm’s Artificial Intelligence Initiative, advises her clients to document, test, and verify every critical step of the AI development process. The purpose is to have a verifiable story—an explanation—to give regulators, investors, and other stakeholders on how the AI system works. The story may never need to be told, but having it, Tonsager advises, should be part of the development protocol.
Final Notes
The Academy achieved something important—it narrowed the knowledge gap between the AI community and the rest of us. Moreover, it did it by creating a learning experience that was as immersive as reading a great book. A book that takes you on a journey where some things are familiar and others fantastical. A book that makes you think about what you have learned days, weeks, and months after it’s been read. A book that prompts you to ask questions you thought you knew the answers to, and ask new questions you would have never thought to ask.
Back to Berkeley Boosts.
For more photos of the program, click here.
If this program sounds interesting, join us for the next AI Unlocked Academy and earn a certificate in Artificial Intelligence from UC Berkeley!
[button url=”https://executive.law.berkeley.edu/in-person-programs/ai-unlocked/” color=”medalist” target=”_blank”]LEARN MORE[/button]